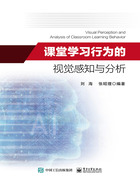
参考文献
[1]新华社. 中共中央关于制定国民经济和社会发展第十四个五年规划和二〇三五年远景目标的建议[S].2020-11.
[2]刘和海,戴濛濛. “互联网+”时代个性化学习实践路径:从“因材施教”走向“可因材施教”[J].中国电化教育,2019(07):46-53.
[3]陈玲,刘静,余胜泉. 个性化在线教育公共服务推进过程中的关键问题思考——对北京市中学教师开放型在线辅导计划的实践反思[J].中国电化教育,2019(11):80-90.
[4]黄荣怀,周伟,杜静,等. 面向智能教育的三个基本计算问题[J].开放教育研究,2019,25(05):11-22.
[5]中华人民共和国教育部. 《教育信息化2. 0行动计划》[S]. 2018-04.
[6]中共中央、国务院. 《中国教育现代化2035》[S]. 2019-02.
[7]浙江省教育厅. 《浙江省教育领域数字化改革工作方案》[S]. 2021-03.
[8]中华人民共和国教育部. 关于加强网络学习空间建设与应用的指导意见[S].2019-01.
[9]教育部等六部门. 关于推进教育新型基础设施建设构建高质量教育支撑体系的指导意见[S].2021-07.
[10]中共中央办公厅、国务院办公厅. 关于进一步减轻义务教育阶段学生作业负担和校外培训负担的意见[S].2021-07.
[11]教育部办公厅. 关于成立校外教育培训监管司的通知[S].2021-06.
[12]教育部办公厅. 关于印发《国家智慧教育公共服务平台接入管理规范(试行)》的通知[S].2022-07.
[13]中共中央、国务院. 《深化新时代教育评价改革总体方案》[S]. 2020-10.
[14]欧盟. 《数字教育行动计划(2021—2027年)》[EB/OL]. 2020-09.
[15]欧盟. 《教育培训数字化转型的关键点》[EB/OL]. 2022-07.
[16]美国高等教育信息化协会. 《2022地平线报告:教与学版》[S]. 2022-04.
[17]王敏. 英国《教育技术战略:释放技术在教育中的潜力》探析[J].世界教育信息,2019,32(17):21-27.
[18]英国政府. 《国家网络安全战略:2022—2030年》[S]. 2021-12.
[19]韩国教育部. 《2022年教育信息化实施计划》[S]. 2022-02.
[20]STANLEY D. Measuring attention using Microsoft Kinect[D]. UK:University of Birmingham, 2013.
[21]ASHWIN T S, JOSE J, RAGHU G, et al. An e-learning system with multifacial emotion recognition using supervised machine learning[C]. 2015 IEEE seventh international conference on technology for education (T4E). IEEE, 2015:23-26.
[22]CHEN J, LUO N, LIU Y Y, et al. A hybrid intelligence-aided approach to affect-sensitive e-learning[J]. Computing, 2016, 98(1):215-233.
[23]杨金朋,薛耀锋,李佳璇,等. 基于人脸表情识别的在线学习情感计算研究[J].中国教育技术装备,2017,18(35):35-43.
[24]WANG L Y. Attention decrease detection based on video analysis in e-learning[M]. Transactions on Edutainment XIV. Springer, Berlin, Heidelberg, 2018:166-179.
[25]贾鹂宇,张朝晖,赵小燕,等. 基于人工智能视频处理的课堂学生状态分析[J].现代教育技术,2019,29(12):82-88.
[26]魏艳涛,秦道影,胡佳敏,等. 基于深度学习的学生课堂行为识别[J].现代教育技术,2019,29(07):87-91.
[27]REVADEKAR A, OAK S, GADEKAR A, et al. Gauging attention of students in an e-learning environment[C]. 2020 IEEE 4th Conference on Information&Communication Technology (CICT). IEEE, 2020:1-6.
[28]王泽杰,沈超敏,赵春,等. 融合人体姿态估计和目标检测的学生课堂行为识别[J].华东师范大学学报(自然科学版),2022(02):55-66.
[29]陈藩,施一萍,胡佳玲,等. 基于卷积神经网络的学生课堂疲劳检测算法[J].传感器与微系统,2022,41(06):153-156.
[30]STANLEY D. Measuring attention using Microsoft Kinect[D]. UK:Rochester Institute of Technology, 2013.
[31]ASHWIN T S, JOSE J, RAGHU G, et al. An e-learning system with multifacial emotion recognition using supervised machine learning[C]. 2015 IEEE seventh international conference on technology for education (T4E). IEEE, 2015:23-26.
[32]CHEN J, LUO N, LIU Y, et al. A hybrid intelligence-aided approach to affect-sensitive e-learning[J]. Computing, 2016, 98(1):215-233.
[33]杨金朋,薛耀锋,李佳璇,等. 基于人脸表情识别的在线学习情感计算研究[J].中国教育技术装备,2017(18):35-36+43.
[34]WANG L. Attention decrease detection based on video analysis in e-learning[M]. Transactions on Edutainment XIV. Springer, Berlin, Heidelberg, 2018:166-179.
[35]贾鹂宇,张朝晖,赵小燕,等. 基于人工智能视频处理的课堂学生状态分析[J].现代教育技术,2019,29(12):82-88.
[36]魏艳涛,秦道影,胡佳敏,等. 基于深度学习的学生课堂行为识别[J].现代教育技术,2019,29(07):87-91.
[37]REVADEKAR A, OAK S, GADEKAR A, et al. Gauging attention of students in an e-learning environment[C]. 2020 IEEE 4th Conference on Information&Communication Technology (CICT). IEEE, 2020:1-6.
[38]王泽杰,沈超敏,赵春,等. 融合人体姿态估计和目标检测的学生课堂行为识别[J].华东师范大学学报(自然科学版),2022(02):55-66.
[39]陈藩,施一萍,胡佳玲,等. 基于卷积神经网络的学生课堂疲劳检测算法[J].传感器与微系统,2022,41(06):153-156.
[40]MOLLAHOSSEINI A, CHAN D, MAHOOR M H. Going Deeper in Facial Expression Recognition using Deep NeuralNetworks[C]. 2016 IEEE Winter Conference on Applications of Computer Vision (WACV). IEEE, 2016:1-10.
[41]王晓峰,王昆,刘轩,等. 自适应重加权池化深度多任务学习的表情识别[J].计算机工程与设计,2022,43(04):1111-1120.
[42]WANG K, PENG X, YANG J, et al. Suppressing uncertainties for large-scale facial expression recognition[C]. Proceedings of the IEEE/CVF Conference on Computer Vision and Pattern Recognition. 2020:6897-6906.
[43]LI H T, SUI M Z, ZHAO F, et al. MVT:Mask vision transformer for facial expression recognition in the wild[D]. Hefei:University of Science and Technology of China. arXiv:2106. 04520, 2022.
[44]CHEN S, WANG J, CHEN Y, et al. Label distribution learning on auxiliary label space graphs for facial expression recognition[C]. Proceedings of the IEEE/CVF Conference on Computer Vision and Pattern Recognition. 2020:13984-13993.
[45]FENG D, REN F. Dynamic facial expression recognition based on two-stream-cnn with lbp-top[C]. 2018 5th IEEE International Conference on Cloud Computing and Intelligence Systems (CCIS). IEEE, 2018:355-359.
[46]LIU D, ZHANG H, Zhou P. Video-based facial expression recognition using graph convolutional networks[C]. 2020 25th International Conference on Pattern Recognition (ICPR). IEEE, 2021:607-614.
[47]ZHAO Z, LIU Q. Former-DFER:Dynamic Facial Expression Recognition Transformer[C]. Proceedings of the 29th ACM International Conference on Multimedia. 2021:1553-1561.
[48]XUE F, TAN Z, ZHU Y, et al. Coarse-to-fine cascaded networks with smooth predicting for video facial expression recognition[C]. Proceedings of the IEEE/CVF Conference on Computer Vision and Pattern Recognition. 2022:2412-2418.
[49]XIA X, YANG L, WEI X, et al. A multi-scale multi-attention network for dynamic facial expression recognition[J]. Multimedia Systems, 2022, 28(2):479-493.
[50]YU M, LIN Y, TANG X, et al. An easy iris center detection method for eye gaze tracking system[J]. Journal of Eye Movement Research, 2015, 8(3).
[51]KANG J J, EIZENMAN M, GUESTRIN E D, et al. Investigation of the cross-ratios method for point-of-gaze estimation[J]. IEEE Transactions on Biomedical Engineering, 2008, 55(9):2293-2302.
[52]CHENG H, LIU Y, FU W, et al. Gazing point dependent eye gaze estimation[J]. Pattern Recognition, 2017, 71:36-44.
[53]LI S, ZHANG X, WEBB J D. 3-D-gaze-based robotic grasping through mimicking human visuomotor function for people with motion impairments[J]. IEEE Transactions on Biomedical Engineering, 2017, 64(12):2824-2835.
[54]ALNAJAR F, GEVERS T, VALENT R, et al. Calibration-free gaze estimation using human gaze patterns[C]. Proceedings of the IEEE international conference on computer vision. 2013:137-144.
[55]LANDER C, GEHRING S, LOCHTEFELD M, et al. Eyemirror:Mobile calibration-free gaze approximation using corneal imaging[C]. Proceedings of the 16th International Conference on Mobile and Ubiquitous Multimedia. 2017:279-291.
[56]WANG K, QIANG J. 3D gaze estimation without explicit personal calibration. Pattern Recognition[J], 2018, 79:216-227.
[57]SUGANO Y, MATSUSHITA Y, SATO Y. Appearance-based gaze estimation using visual saliency[J]. IEEE transactions on pattern analysis and machine intelligence, 2012, 35(2):329-341.
[58]PERRA D, GUPTA R K, FRAHM J M. Adaptive eye-camera calibration for head-worn devices[C]. 2015 IEEE Conference on Computer Vision and Pattern Recognition (CVPR), 2015:4146-4155.
[59]ZHANG X, SUGANO Y, FRITZ M, et al. Appearance-based gaze estimation in the wild[C]. 2015 IEEE Conference on Computer Vision and Pattern Recognition (CVPR), 2015:4511-4520.
[60]ZHANG X, SUGANO Y, FRITZ M, et al. Mpiigaze:Real-world dataset and deep appearance-based gaze estimation[J]. IEEE transactions on pattern analysis and machine intelligence, 2017, 41(1):162-175.
[61]CHEN Z, SHI B E. Appearance-based gaze estimation using dilated-convolutions[C]. Asian Conference on Computer Vision. Springer, Cham, 2018:309-324.
[62]FISCHER T, JIN CHANG H, DEMIRIS Y. Rt-gene:Real-time eye gaze estimation in natural environments[C]. Proceedings of the European conference on computer vision (ECCV), 2018:334-352.
[63]CHENG Y, LU F, ZHANG X. Appearance-based gaze estimation via evaluation-guided asymmetric regression[C]. Proceedings of the European Conference on Computer Vision (ECCV), 2018:100-115.
[64]CHENG Y, HUANG S, WANG F, et al. A coarse-to-fine adaptive network for appearance-based gaze estimation[C]. Proceedings of the AAAI Conference on Artificial Intelligence, 2020, 34(07):10623-10630.
[65]BAO Y, CHENG Y, LIU Y, et al. Adaptive feature fusion network for gaze tracking in mobile tablets[C]. 2020 25th International Conference on Pattern Recognition (ICPR). IEEE, 2021:9936-9943.
[66]SUN Y, WANG X, TANG X. Deep convolutional network cascade for facial point detection[C]. Proceedings of the IEEE conference on computer vision and pattern recognition, 2013:3476-3483.
[67]闵秋莎,刘能,陈雅婷,等. 基于面部特征点定位的头部姿态估计[J].计算机工程,2018,44(06):263-269.
[68]XIN M, MO S, LIN Y. EVA-GCN:Head pose estimation based on graph convolutional networks[C]. Proceedings of the IEEE/CVF Conference on Computer Vision and Pattern Recognition. 2021:1462-1471.
[69]陈国军,杨静,程琰,等. 基于RGBD的实时头部姿态估计[J].图学学报,2019,40(04):681-688.
[70]XU Y, JUNG C, CHANG Y. Head pose estimation using deep neural networks and 3D point clouds[J]. Pattern Recognition, 2022, 121:108-210.
[71]MASSIMILIANO P, ANGELO C. Head pose estimation in the wild using convolutional neural networks and adaptive gradient methods[J]. Pattern Recognition, 2017, 71:132-143.
[72]LIU H, NIE H, ZHANG Z, et al. Anisotropic angle distribution learning for head pose estimation and attention understanding in human-computer interaction[J]. Neurocomputing, 2021, 433:310-322.
[73]LIU H, FANG S, ZHANG Z, et al. MFDNet:Collaborative poses perception and matrix Fisher distribution for head pose estimation[J]. IEEE Transactions on Multimedia, 2021, 24:2449-2460.
[74]齐永锋,马中玉. 基于深度残差网络的多损失头部姿态估计[J].计算机工程,2020,46(12):247-253.
[75]RPBERTO V, JOSE M B, LUIS B. Multi-task head pose estimation in-the-wild[J]. IEEE Transactions on Pattern Analysis and Machine Intelligence, 2020, 43(8):2874-2881.
[76]NAINA D. LwPosr:Lightweight Efficient Fine Grained Head Pose Estimation[C]. Proceedings of the IEEE/CVF Winter Conference on Applications of Computer Vision. 2022:1495-1505.
[77]KRIZHEVSKY A, SUTSKEVER I, HINTON G E. Imagenet classification with deep convolutional neural networks[J]. Communications of the ACM, 2017, 60. 6:84-90.
[78]TOSHEV A, SZEGEDY C. Deeppose:Human pose estimation via deep neural networks[C]. In:Proceedings of the IEEE conference on computer vision and pattern recognition, 2014:1653-1660.
[79]WEI S E, RAMAKRISHNA V, KANADE T, et al. Convolutional pose machines[C]. Proceedings of the IEEE conference on Computer Vision and Pattern Recognition, 2016:4724-4732.
[80]NEWELL A, YANG K Y, DENG J. Stacked hourglass networks for human pose estimation[C]. European conference on computer vision. Springer, Cham, 2016:483-499.
[81]CHEN Y L, WANG Z H, PENG Y X, et al. Cascaded pyramid network for multi-person pose estimation[C]. Proceedings of the IEEE conference on computer vision and pattern recognition, 2018:7103-7112.
[82]PISHCHULIN L, INSAFUTDINOV E, TANG S Y, et al. Deepcut:Joint subset partition and labeling for multi person pose estimation[C]. Proceedings of the IEEE Conference on Computer Vision and Pattern Recognition, 2016:4929-4937.
[83]CAO Z, HIDALGO G, SIMON T, et al. OpenPose:Realtime multi-person 2D pose estimation using part affinity fields[J]. Proceedings of the IEEE Transactions on Pattern Analysis and Machine Intelligence, 2021:172-186.
[84]KREISS S, BERTONI L, ALAHI A. OpenPifPaf:Composite Fields for Semantic Keypoint Detection and Spatio-Temporal Association[C]. Proceedings of the IEEE Transactions on Intelligent Transportation Systems, 2021.
[85]NEWELL A, HUANG Z A, DENG J. Associative embedding:End-to-end learning for joint detection and grouping[J]. Advances in Neural Information Processing Systems, volume 30. Curran Associates, Inc. , 2017(30):2278-2288.
[86]NIE X C, FENG J S, ZHANG J F, et al. Single-stage multi-person pose machines[C]. Proceedings of the IEEE/CVF International Conference on Computer Vision, 2019:6951-6960.
[87]ZHOU X Y, WANG D Q, KRAHENBUHL P. Objects as points[C]. arXiv preprint, 2019.
[88]MAO W A, TIAN Z, WANG X L. Fcpose:Fully convolutional multi-person pose estimation with dynamic instance-aware convolutions[C]. Proceedings of the IEEE/CVF Conference on Computer Vision and Pattern Recognition, 2021:9034-9043.
[89]SHI D H, WEI X, YU X D, et al. Inspose:Instance-aware networks for single-stage multi-person pose estimation[C]. In Proceedings of the 29th ACM International Conference on Multimedia, 2021:3079-3087.
[90]XUE N, WU T F, XIA G S, et al. Learning Local-Global Contextual Adaptation for Multi-Person Pose Estimation[C]. Proceedings of the IEEE Conference on Computer Vision and Pattern Recognition, 2022:13065-13074.
[91]VASWANI A, SHAZEER N, PARMAR N, et al. Attention is all you need[C]. Proceedings of the 31st International Conference on Neural Information Processing Systems, 2017:6000-6010.
[92]LI K, WANG S J, ZHANG X, et al. Pose recognition with cascade transformers[C]. Proceedings of the IEEE/CVF Conference on Computer Vision and Pattern Recognition, 2021:1944-1953.
[93]SHI D H, WEI X, LI L Q, et al. End-to-End Multi-Person Pose Estimation with Transformers[C]. Proceedings of the IEEE Conference on Computer Vision and Pattern Recognition, 2022:11069-11078.
[94]LI Y J, ZHANG S K, WANG Z C, et al. Tokenpose:Learning keypoint tokens for human pose estimation[C]. Proceedings of the IEEE/CVF International Conference on Computer Vision, 2021:11313-11322.
[95]YANG S, QUAN Z B, NIE M, et al. Transpose:Keypoint localization via transformer[C]. Proceedings of the IEEE/CVF International Conference on Computer Vision, 2021:11802-11812.
[96]王一岩,郑永和. 多模态数据融合:破解智能教育关键问题的核心驱动力[J].现代远程教育研究,2022,34(02):93-102.