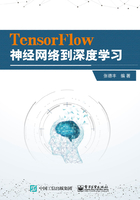
3.3 激活函数
激活函数的主要作用就是用来加入非线性因素的,以解决线性模型表达能力不足的缺陷,在整个神经网络里起到至关重要的作用。
因为神经网络的数学基础是处处可微的,所以选取的激活函数要能保证数据输入与输出也是可微的。
在神经网络里常用的激活函数有sigmoid、tanh和relu等。
3.3.1 sigmoid激活函数
sigmoid激活函数也被称为S型函数,它可以将整个实数区间映射到(0,1)区间,因此经常被用来计算概率,它也是在传统神经网络中被经常使用的一种激活函数。
sigmoid激活函数由下列公式定义:

sigmoid激活函数曲线如图3-6所示,其中,x可以是正无穷到负无穷,但是对应的y却在0~1的区间内,所以,经过sigmoid激活函数输出的函数都会落在0~1的区间内,即sigmoid激活函数能够把输入的值“压缩”到0~1。

图3-6 sigmoid激活函数曲线
例如:

运行程序,输出如下:

可总结出sigmoid激活函数的优缺点如下所述。
sigmoid激活函数的优点:输出的映射区间(0,1)内单调连续,非常适合用在输出层,并且比较容易求导。
sigmoid激活函数的缺点:它具有软饱和性,即当输入x趋向于无穷的时候,它的导数会趋于0,导致很容易产生梯度消失。
此外,在TensorFlow中,提供了log_sigmoid函数用于对sigmoid激活函数求log,它将整个实数区间映射到了(负无穷,0)。
【例3-1】利用log_sigmoid函数对给定的sigmoid激活函数求log。


运行程序,输出如下,log_sigmoid函数图像如图3-7所示。


图3-7 log_sigmoid函数图像
3.3.2 tanh激活函数
tanh激活函数是双曲正切函数,它将整个实数区间映射到了(-1,1),tanh激活函数也具有软饱和性。它的输出以0为中心,tanh激活函数的收敛速度比sigmoid激活函数的收敛速度要快,由于存在软饱和性,所以tanh激活函数也存在梯度消失的问题。
tanh激活函数由下列公式定义:

【例3-2】绘制tanh激活函数曲线。

运行程序,输出如下,tanh激活函数曲线如图3-8所示。



图3-8 tanh激活函数曲线
由图3-8可以看出,其x取值也是从正无穷到负无穷,对应的y值变为-1~1,相对于sigmoid激活函数有更广的值域。但也显而易见,tanh激活函数与sigmoid激活函数有一样的缺陷,也是饱和问题,所以在使用tanh激活函数时,要注意输入值的绝对值不能过大,否则模型无法训练。
3.3.3 relu激活函数
除了sigmoid激活函数和tanh激活函数,还有一个更为常用的relu激活函数(也称为Rectifier)。其由以下公式定义:

该式非常简单,大于0的留下,否则一律为0。relu激活函数应用的广泛性与它的优势是分不开的,这种对正向信号的重视,忽略了负向信号的特性,与我们人类神经元细胞对信号的反映极其相似。所以在神经网络中取得了很好的拟合效果。
另外,由于relu激活函数运算简单,大大提升了机器的运动效率,也是relu激活函数一个很大的优点。
【例3-3】绘制relu激活函数曲线。

运行程序,输出如下,relu激活函数曲线图如图3-9所示。


图3-9 relu激活函数曲线图
与relu激活函数类似的还有softplus激活函数,它们二者的区别在于:softplus激活函数会更加平滑,但计算量很大,而且对于小于0的值保留的相对更多一些。其定义公式为

【例3-4】绘制softplus激活函数曲线。

运行程序,输出如下,softplus激活函数曲线图如图3-10所示。


图3-10 softplus激活函数曲线图
虽然,relu激活函数在信号响应上有很多优势,但这仅仅在正向传播方面。由于其对负值的全部舍去,因此很容易使模型输出为全零,从而无法再进行训练。例如,随机初始化的w值中有一个值是负值,其对应的正值输入值特征也就全部被屏蔽了,同理,对应负值输入值反而被激活了。这显然不是我们想要的结果。于是在基于relu激活函数的基础上又演化出了一些变种函数。
·relu6激活函数:定义min(max(features,0),6),也就是说它的取值区间被限定为[0,6]。
【例3-5】绘制relu6激活函数曲线。

运行程序,输出如下,relu6激活函数曲线图如图3-11所示。


图3-11 relu6激活函数曲线图
·crelu激活函数:定义为[relu(x),relu(-x)]相对于relu(x),crelu激活函数的输出会增加一倍。
【例3-6】利用crelu激活函数对输入数据进行计算。

运行程序,输出如下:

·leak_relu激活函数:leak_relu激活函数会给x<0一个斜率,而不是将所有x<0都输出0,默认斜率是0.2,x>0的部分仍然输出是x,即斜率为1,保持不变。
【例3-7】绘制leak_relu激活函数曲线。


运行程序,输出如下,leak_relu激活函数曲线图如图3-12所示。


图3-12 leak_relu激活函数曲线图
3.3.4 dropout激活函数
dropout激活函数会以概率keep_prob来决定神经元是否被抑制。如果被抑制,该神经元则输出为0;如果不被抑制,该神经元则输出为输入的1/keep_prob倍,每个神经元是否会被抑制是相互独立的。神经元是否被抑制还可以通过noise_shape来调节,当noise_shape[i]=shape(x)[i]时,x中的元素是相互独立的。如果shape(x)=[k,l,m,n](k表示数据的个数,l表示数据的行数,m表示数据的列数,n表示通道数),当noise_shape=[k,l,l,n]时,表示数据的个数与通道是相互独立的,但是与数据的行和列是有关联的,即要么都为0,要么都为输入的1/keep_prob倍。
【例3-8】利用dropout激活函数对输入随机数进行计算。


运行程序,输出如下:
