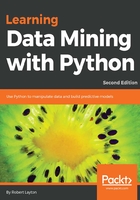
Algorithms for affinity analysis
We introduced a basic method for affinity analysis in Chapter 1, Getting Started with Data Mining, which tested all of the possible rule combinations. We computed the confidence and support for each rule, which in turn allowed us to rank them to find the best rules.
However, this approach is not efficient. Our dataset in Chapter 1, Getting Started with Data Mining, had just five items for sale. We could expect even a small store to have hundreds of items for sale, while many online stores would have thousands (or millions!). With a naive rule creation, such as our previous algorithm from Chapter 1, Getting Started with Data Mining, the growth in the time needed to compute these rules increases exponentially. As we add more items, the time it takes to compute all rules increases significantly faster. Specifically, the total possible number of rules is 2n - 1. For our five-item dataset, there are 31 possible rules. For 10 items, it is 1023. For just 100 items, the number has 30 digits. Even the drastic increase in computing power couldn't possibly keep up with the increases in the number of items stored online. Therefore, we need algorithms that work smarter, as opposed to computers that work harder.
The classic algorithm for affinity analysis is called the Apriori algorithm. It addresses the exponential problem of creating sets of items that occur frequently within a database, called frequent itemsets. Once these frequent itemsets are discovered, creating association rules is straightforward, which we will see later in the chapter.
The intuition behind Apriori is both simple and clever. First, we ensure that a rule has sufficient support within the dataset. Defining a minimum support level is the key parameter for Apriori. To build a frequent itemset we combine smaller frequent itemsets. For itemset (A, B) to have a support of at least 30, both A and B must occur at least 30 times in the database. This property extends to larger sets as well. For an itemset (A, B, C, D) to be considered frequent, the set (A, B, C) must also be frequent (as must D).
These frequent itemsets can be built and possible itemsets that are not frequent (of which there are many) will never be tested. This saves significant time in testing new rules, as the number of frequent itemsets is expected to be significantly fewer than the total number of possible itemsets.
Other example algorithms for affinity analysis build on this, or similar concepts, including the Eclat and FP-growth algorithms. There are many improvements to these algorithms in the data mining literature that further improve the efficiency of the method. In this chapter, we will focus on the basic Apriori algorithm.