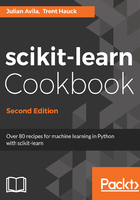
What this book covers
Chapter 1, High-Performance Machine Learning – NumPy, features your first machine learning algorithm with support vector machines. We distinguish between classification (what type?) and regression (how much?). We predict an outcome on data we have not seen.
Chapter 2, Pre-Model Workflow and Pre-Processing, exposes a realistic industrial setting with plenty of data munging and pre-processing. To do machine learning, you need good data, and this chapter tells you how to get it and get it into good form for machine learning.
Chapter 3, Dimensionality Reduction, discusses reducing the number of features to simplify machine learning and allow better use of computational resources.
Chapter 4, Linear Models with scikit-learn, tells the story of linear regression, the oldest predictive model, from the machine learning and artificial intelligence lenses. You deal with correlated features with ridge regression, eliminate related features with LASSO and cross-validation, or eliminate outliers with robust median-based regression.
Chapter 5, Linear Models – Logistic Regression, examines the important healthcare datasets for cancer and diabetes with logistic regression. This model highlights both similarities and differences between regression and classification, the two types of supervised learning.
Chapter 6, Building Models with Distance Metrics, places points in your familiar Euclidean space of school geometry, as distance is synonymous with similarity. How close (similar) or far away are two points? Can we group them together? With Euclid's help, we can approach unsupervised learning with k-means clustering and place points in categories we do not know in advance.
Chapter 7, Cross-Validation and Post-Model Workflow, features how to select a model that works well with cross-validation: iterated training and testing of predictions. We also save computational work with the pickle module.
Chapter 8, Support Vector Machines, examines in detail the support vector machine, a powerful and easy-to-understand algorithm.
Chapter 9, Tree Algorithms and Ensembles, features the algorithms of decision making: decision trees. This chapter introduces meta-learning algorithms, diverse algorithms that vote in some fashion to increase overall predictive accuracy.
Chapter 10, Text and Multiclass Classification with scikit-learn, reviews the basics of natural language processing with the simple bag-of-words model. In general, we view classification with three or more categories.
Chapter 11, Neural Networks, introduces a neural network and perceptrons, the components of a neural network. Each layer figures out a step in a process, leading to a desired outcome. As we do not program any steps specifically, we venture into artificial intelligence. Save the neural network so that you can keep training it later, or load it and utilize it as part of a stacking ensemble.
Chapter 12, Create a Simple Estimator, helps you make your own scikit-learn estimator, which you can contribute to the scikit-learn community and take part in the evolution of data science with scikit-learn.
Preface
Starting with installing and setting up scikit-learn, this book contains highly practical recipes on common supervised and unsupervised machine learning concepts. Acquire your data for analysis; select the necessary features for your model; and implement popular techniques such as linear models, classification, regression, clustering, and more in no time at all! The book also contains recipes on evaluating and fine-tuning the performance of your model. The recipes contain both the underlying motivations and theory for trying a technique, plus all the code in detail.
- Donald Knuth
scikit-learn and Python allow fast prototyping, which is in a sense the opposite of Donald Knuth's premature optimization. Personally, scikit-learn has allowed me to prototype what I once thought was impossible, including large-scale facial recognition systems and stock market trading simulations. You can gain instant insights and build prototypes with scikit-learn. Data science is, by definition, scientific and has many failed hypotheses. Thankfully, with scikit-learn you can see what works (and what does not) within the next few minutes.
Additionally, Jupyter (IPython) notebooks feature a nice interface that is very welcoming to beginners and experts alike and encourages a new scientific software engineering mindset. This welcoming nature is refreshing because, in innovation, we are all beginners.
In the last chapter of this book, you can make your own estimator and Python transitions from a scripting language to more of an object-oriented language. The Python data science ecosystem has the basic components for you to make your own unique style and contribute heavily to the data science team and artificial intelligence.
In analogous fashion, algorithms work as a team in the stacker. Diverse algorithms of different styles vote to make better predictions. Some make better choices than others, but as long as the algorithms are different, the choice in the end will be the best. Stackers and blenders came to prominence in the Netflix $1 million prize competition won by the team Pragmatic Chaos.
Welcome to the world of scikit-learn: a very powerful, simple, and expressive machine learning library. I am truly excited to see what you come up with.