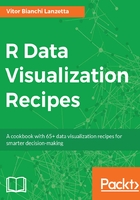
上QQ阅读APP看书,第一时间看更新
How to do it...
- Load ggplot2 and call for the geom_smooth() layer:
> library(ggplot2)
> scatter <- ggplot(data = iris,
aes(x = Petal.Length, y = Petal.Width)) +
geom_point(alpha = .5, aes(colour = Species, shape = Species))
> scatter +
geom_smooth(method = 'lm', se = F, show.legend = F,
aes(group = Species))
Resulting illustration would look like the following figure 2.11:

Figure 2.11 - scatterplot with grouped regression lines.
- For ggvis, call layer_model_predictions() along with the group_by() function:
> library(ggvis)
> ggvis( iris, x = ~Petal.Length, y = ~Petal.Width, opacity := .5) %>%
> layer_points( shape = ~Species, fill = ~Species) %>% group_by(Species) %>%
> layer_model_predictions( model = 'lm', stroke = ~Species)
- Using plotly, combine add_lines() with the lm() function to achieve a similar result:
> library(plotly)
> plot_ly(iris, x = ~Petal.Length, y = ~Petal.Width,
showlegend = F, alpha = .5, color = ~Species) %>%
add_markers(showlegend = T, symbol = ~Species) %>%
add_lines(data = iris %>% filter(Species == 'setosa'),
y = ~fitted(lm( Petal.Width ~ Petal.Length))) %>%
add_lines(data = iris %>% filter(Species == 'versicolor'),
y = ~fitted(lm( Petal.Width ~ Petal.Length))) %>%
add_lines(data = iris %>% filter(Species == 'virginica'),
y = ~fitted(lm( Petal.Width ~ Petal.Length)))
Following section holds some explanations.