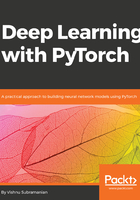
上QQ阅读APP看书,第一时间看更新
ReLU
ReLU has become more popular in the recent years; we can find either its usage or one of its variants' usages in almost any modern architecture. It has a simple mathematical formulation:
f(x)=max(0,x)
In simple words, ReLU squashes any input that is negative to zero and leaves positive numbers as they are. We can visualize the ReLU function as follows:

Image source: http://datareview.info/article/eto-nuzhno-znat-klyuchevyie-rekomendatsii-po-glubokomu-obucheniyu-chast-2/
Some of the pros and cons of using ReLU are as follows:
- It helps the optimizer in finding the right set of weights sooner. More technically it makes the convergence of stochastic gradient descent faster.
- It is computationally inexpensive, as we are just thresholding and not calculating anything like we did for the sigmoid and tangent functions.
- ReLU has one disadvantage; when a large gradient passes through it during the backward propagation, they often become non-responsive; these are called dead neutrons, which can be controlled by carefully choosing the learning rate. We will discuss how to choose learning rates when we discuss the different ways to adjust the learning rate in Chapter 4, Fundamentals of Machine Learning.