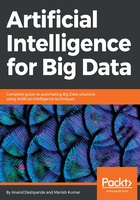
Least square method
Let's consider the same training data we referred to earlier in this chapter. We have values for the independent variable, x, and corresponding values for the dependent variable, y. These values are plotted on a two-dimensional scatter plot. The goal is to draw a regression line through the training data so as to minimize the error of our predictions. The linear regression line with minimum error always passes the mean intercept for x and y values.
The following figure shows the least square method:
Figure 3.8 Least square method
The formula for calculating the y intercept is as follows:
The least square method calculates the y intercept and the slope of the line with the following steps:
- Calculate the mean of all the x values (119.33).
- Calculate the mean of all the y values (303.20).
- Calculate difference from the mean for all the x and y values.
- Calculate the square of mean difference for all the x values.
- Multiply the mean difference of x by the mean difference of y for all the combinations of x and y.
- Calculate the sum squares of all the mean differences of the x values (56743.33).
- Calculate the sum of mean difference products of the x and y values (90452.00).
- The slope of the regression line is obtained by piding the sum of the mean difference products of x and y by the sum of the squares of all the mean differences of the x values (90452.00 / 56743.33 = 1.594). In this training data, since there is direct proportion between the x and y values, the slope is positive. This is the value for b in our equation.
- We need to calculate the value of the y intercept (a) by solving the following equation, y = a + 1.594 * x.
Remember, the regression line always passes through the mean intercept of the x and y values.
- Therefore, 303.2 = a + (1.594 * 119.33).
- Solving this, we get a = 112.98 as the y intercept for the regression line.
At this point, we have created our regression line with which we can predict the value of the dependent variable, y, for a value of x. We need to see how close our regression line mathematically is to the actual data points. We will use one of the most popular statistical techniques, R-squared, for this purpose. It is also called the coefficient of determination. R-squared calculates the % of response variable variation for the linear regression model we have developed. R-squared values will always be between 0% and 100%. A higher value of R-squared indicates that the model fits the training data well; generally termed the goodness of fit. The following diagram shows the calculation of R-squared with some sample data points:
Figure 3.9 Calculation of R-squared
Let's use our training data to calculate R-squared based on the formula in the preceding image. Please refer to the diagram we just saw, in this case, R-squared = 144175.50 / 156350.40 = 0.9221. This value is an indication that the model is fitting the training data very well. There is another parameter we can derive, called standard error, from the estimate. This is calculated as:
In this formula, n is the sample size or the number of observations. With our dataset, the standard error of the estimate comes out to be 30.59.
Let's calculate the R-squared for our training dataset with the Spark machine learning library:
import org.apache.spark.ml.feature.LabeledPoint
import org.apache.spark.ml.linalg.Vectors
import org.apache.spark.ml.regression.LinearRegression
val linearRegrsssionSampleData = sc.textFile("aibd/linear_regression_sample.txt")
val labeledData = linearRegrsssionSampleData.map { line =>
val parts = line.split(',')
LabeledPoint(parts(0).toDouble, Vectors.dense(parts(1).toDouble))
}.cache().toDF
val lr = new LinearRegression()
val model = lr.fit(labeledData)
val summary = model.summary
println("R-squared = "+ summary.r2)
This program produces the following output. Note the same value for R-squared: