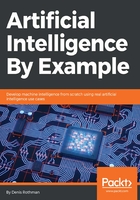
Apply Machine Thinking to a Human Problem
In the first chapter, the MDP reinforcement program produced a result as an output matrix. In Chapter 2, Think Like a Machine, the McCulloch-Pitts system of neurons produced an input reward matrix. However, the intermediate or final results of these two functions need to be constantly measured. Good measurement solves a substantial part of a given problem since decisions rely on them. Reliable decisions are made with reliable evaluations. The goal of this chapter is to introduce measurement methods.
The key function of human intelligence, decision-making, relies on the ability to evaluate a situation. No decision can be made without measuring the pros and cons and factoring the parameters.
Mankind takes great pride in its ability to evaluate. However, in many cases, a machine can do better. Chess represents the pride of mankind in thinking strategy. A chessboard is often present in many movies to symbolize human intelligence.
Today, not a single chess player can beat the best chess engines. One of the extraordinary core capacities of a chess engine is the evaluation function; it takes many parameters into account more precisely than humans.
This chapter focuses on the main concepts of evaluation and measurement; they set the path to deep learning gradient descent-driven models, which will be explained in the following chapter.
The following topics will be covered in this chapter:
- Evaluation of the episodes of a learning session
- Numerical convergence measurements
- An introduction to the idea of cross-entropy convergence
- Decision tree supervised learning as an evaluation method
- Decision tree supervised learning as a predictive model
- How to apply evaluation tools to a real-life problem you build on your own