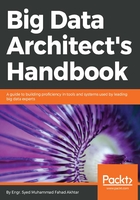
Veracity
This vector deals with the uncertainty of data. It may be because of poor data quality or because of the noise in data. It's human behavior that we don't trust the information provided. This is one of the reasons that one in three business leaders don't trust the information they use for making decisions.
We can consider in a way that velocity and variety are dependent on the clean data prior to analysis and making decisions, whereas veracity is the opposite to these characteristics as it is derived from the uncertainty of data. Let's take the example of apples, where you have to decide whether they are of good quality. Perhaps a few of them are average or below average quality. Once you start checking them in huge quantities, perhaps your decision will be based on the condition of the majority, and you will make an assumption regarding the rest, because if you start checking each and every apple, the remaining good-quality ones may lose their freshness. The following diagram is an illustration of the example of apples:

Veracity: illustration of uncertainty in apple example
The main challenge is that you don't get time to clean streaming data or high-velocity data to eliminate uncertainty. Data such as events data is generated by machines and sensors and if you wait to first clean and process it, that data might lose value. So you must process it as is, taking account of uncertainty.
Veracity is all about uncertainty and how much trust you have in your data, but when we use it in terms of the big data context, it may be that we have to redefine trusted data with a different definition. In my opinion, it is the way you are using data or analyzing it to make decisions. Because of the trust you have in your data, it influences the value and impact of the decisions you make.
Let's now look at the fifth characteristic of big data, which is variability.