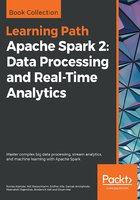
What This Book Covers
Chapter 1, A First Taste and What's New in Apache Spark V2, provides an overview of Apache Spark, the functionality that is available within its modules, and how it can be extended. It covers the tools available in the Apache Spark ecosystem outside the standard Apache Spark modules for processing and storage. It also provides tips on performance tuning.
Chapter 2, Apache Spark Streaming, talks about continuous applications using Apache Spark Streaming. You will learn how to incrementally process data and create actionable insights.
Chapter 3, Structured Streaming, talks about Structured Streaming – a new way of defining continuous applications using the DataFrame and Dataset APIs.
Chapter 4, Apache Spark MLlib, introduces you to MLlib, the de facto standard for machine learning when using Apache Spark.
Chapter 5, Apache SparkML, introduces you to the DataFrame-based machine learning library of Apache Spark: the new first-class citizen when it comes to high performance and massively parallel machine learning.
Chapter 6, Apache SystemML, introduces you to Apache SystemML, another machine learning library capable of running on top of Apache Spark and incorporating advanced features such as a cost-based optimizer, hybrid execution plans, and low-level operator re-writes.
Chapter 7, Apache Spark GraphX, talks about Graph processing with Scala using GraphX. You will learn some basic and also advanced graph algorithms and how to use GraphX to execute them.
Chapter 8, Spark Tuning, digs deeper into Apache Spark internals and says that while Spark is great in making us feel as if we are using just another Scala collection, we shouldn't forget that Spark actually runs in a distributed system. Therefore, throughout this chapter, we will cover how to monitor Spark jobs, Spark configuration, common mistakes in Spark app development, and some optimization techniques.
Chapter 9, Testing and Debugging Spark, explains how difficult it can be to test an application if it is distributed; then, we see some ways to tackle this. We will cover how to do testing in a distributed environment, and testing and debugging Spark applications.
Chapter 10, Practical Machine Learning with Spark Using Scala, covers installing and configuring a real-life development environment with machine learning and programming with Apache Spark. Using screenshots, it walks you through downloading, installing, and configuring Apache Spark and IntelliJ IDEA along with the necessary libraries that would reflect a developer’s desktop in a real-world setting. It then proceeds to identify and list over 40 data repositories with real-world datasets that can help the reader in experimenting and advancing even further with the code recipes. In the final step, we run our first ML program on Spark and then provide directions on how to add graphics to your machine learning programs, which are used in the subsequent chapters.
Chapter 11, Spark’s Three Data Musketeers for Machine Learning - Perfect Together, provides an end-to-end treatment of the three pillars of resilient distributed data manipulation and wrangling in Apache Spark. The chapter comprises detailed recipes covering RDDs, DataFrame, and Dataset facilities from a practitioner’s point of view. Through an exhaustive list of 17 recipes, examples, references, and explanation, it lays out the foundation to build a successful career in machine learning sciences. The chapter provides both functional (code) as well as non-functional (SQL interface) programming approaches to solidify the knowledge base reflecting the real demands of a successful Spark ML engineer at tier 1 companies.
Chapter 12, Common Recipes for Implementing a Robust Machine Learning System, covers and factors out the tasks that are common in most machine learning systems through 16 short but to-the-point code recipes that the reader can use in their own real-world systems. It covers a gamut of techniques, ranging from normalizing data to evaluating the model output, using best practice metrics via Spark’s ML/MLlib facilities that might not be readily visible to the reader. It is a combination of recipes that we use in our day-to-day jobs in most situations but is listed separately to save on space and complexity of other recipes.
Chapter 13, Recommendation Engine that Scales with Spark, covers how to explore your data set and build a movie recommendation engine using Spark’s ML library facilities. It uses a large dataset and some recipes in addition to figures and write-ups to explore the various methods of recommenders before going deep into collaborative filtering techniques in Spark.
Chapter 14, Unsupervised Clustering with Apache Spark 2.0, covers the techniques used in unsupervised learning, such as KMeans, Mixture, and Expectation (EM), Power Iteration Clustering (PIC), and Latent Dirichlet Allocation (LDA), while also covering the why and how to help the reader to understand the core concepts. Using Spark Streaming, the chapter commences with a real-time KMeans clustering recipe to classify the input stream into labeled classes via unsupervised means.
Chapter 15, Implementing Text Analytics with Spark 2.0 ML Library, covers the various techniques available in Spark for implementing text analytics at scale. It provides a comprehensive treatment by starting from the basics, such as Term Frequency (TF) and similarity techniques, such as Word2Vec, and moves on to analyzing a complete dump of Wikipedia for a real-life Spark ML project. The chapter concludes with an in-depth discussion and code for implementing Latent Semantic Analysis (LSA) and Topic Modeling with Latent Dirichlet Allocation (LDA) in Spark.
Chapter 16, Spark Streaming and Machine Learning Library, starts by providing an introduction to and the future direction of Spark streaming and then proceeds to provide recipes for both RDD-based (DStream) and structured streaming to establish a baseline. The chapter then proceeds to cover all the available ML streaming algorithms in Spark at the time of writing this book. The chapter provides code and shows how to implement streaming DataFrame and streaming data sets, and then proceeds to cover queueStream for debugging before it goes into Streaming KMeans (unsupervised learning) and streaming linear models such as Linear and Logistic regression using real-world datasets.