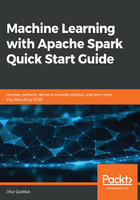
Tasks
Tasks are the smallest unit of execution in Spark, with a single task being executed on one executor; in other words, a single task cannot span multiple executors. All the tasks making up one stage share the same code to be executed, but act on different partitions of the data. The number of tasks that can be processed by an executor is bounded by the number of cores associated with that executor. Therefore, the total number of tasks that can be executed in parallel across an entire Spark cluster can be calculated by multiplying the number of cores per executor by the number of executors. This value then provides a quantifiable measure of the level of parallelism offered by your Spark cluster.
In Chapter 2, Setting Up a Local Development Environment, we will discuss how to install, configure, and administer a single-node standalone Spark cluster for development purposes, as well as discussing some of the basic configuration options exposed by Spark. Then, in Chapter 3, Artificial Intelligence and Machine Learning, and onward, we will take advantage of Spark's machine learning library, MLlib, so that we may employ Spark as a distributed advanced analytics engine. To learn more about Apache Spark, please visit http://spark.apache.org/.