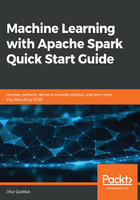
DataFrames
Like RDDs, they are an immutable and distributed collection of records partitioned across the worker nodes in a Spark cluster. However, unlike RDDs, data is organized into named columns conceptually similar to tables in relational databases and tabular data structures found in other programming languages, such as Python and R. Because DataFrames offer the ability to impose a schema on distributed data, they are more easily exposed to more familiar programming languages such as SQL, which makes them a popular and arguably easier data structure to work with and manipulate, as well as being more efficient than RDDs.
The main disadvantage of DataFrames however is that, similar to Spark SQL string queries, analysis errors are only caught at runtime and not during compilation. For example, imagine a DataFrame called df with the named columns firstname, lastname, and gender. Now imagine that we coded the following statement:
df.filter( df.age > 30 )
This statement attempts to filter the DataFrame based on a missing and unknown column called age. Using the DataFrame API, this error would not be caught at compile time but instead only at runtime, which could be costly and time-consuming if the Spark application in question involved multiple transformations and aggregations prior to this statement.