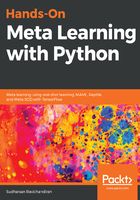
What this book covers
Chapter 1, Introduction to Meta Learning, helps us to understand what meta learning is and covers the different types of meta learning. We will also learn how meta learning uses few-shot learning by learning from a few data points. We will then see how to become familiar with gradient descent. Later in the chapter, we will see optimization as a model for the few shot learning setting.
Chapter 2, Face and Audio Recognition Using Siamese Networks, starts by explaining what siamese networks are and how siamese networks are used in the one-shot learning setting. We will look at the architecture of a siamese network and some of the applications of a siamese network. Then, we will see how to use the siamese networks to build face and audio recognition models.
Chapter 3, Prototypical Networks and Their Variants, explains what prototypical networks are and how they are used in the few shot learning scenario. We will see how to build a prototypical network to perform classification on an omniglot character set. Later in the chapter, we will look at different variants of prototypical networks, such as the Gaussian prototypical networks and semi-prototypical networks.
Chapter 4, Relation and Matching Networks Using TensorFlow, helps us to understand the relation network architecture and how relation network is used in one-shot, few-shot, and zero-shot learning settings. We will then see how to build a relation network using TensorFlow. Next, we will learn about the matching network and its architecture. We will also explore full contextual embeddings and how to build a matching network using TensorFlow.
Chapter 5, Memory-Augmented Neural Networks, covers what neural Turing machines (NTMs) are and how they make use of external memory for storing and retrieving information. We will look at different addressing mechanisms used in NTMs and then we will learn about memory augmented neural networks and how they differ from the NTM architecture.
Chapter 6, MAML and Its Variants, deals with one of the popular meta learning algorithms, called model-agnostic meta learning (MAML). We will explore what MAML is and how it is used in supervised and reinforcement learning settings. We will also see how to build MAML from scratch. Then, we will learn about adversarial meta learning and CAML, which is used for fast context adaptation in meta learning.
Chapter 7, Meta-SGD and Reptile, explain how meta-SGD is used to learn all the ingredients of gradient descent algorithms, such as initial weights, learning rates, and the update direction. We will see how to build meta-SGD from scratch. Later in the chapter, we will learn about the reptile algorithm and see how it serves as an improvement over MAML. We will also see how to use the reptile algorithm for sine wave regression.
Chapter 8, Gradient Agreement as an Optimization Objective, covers how we can use gradient agreement as an optimization objective in the meta learning setting. We will learn what gradient agreement is and how it can enhance meta learning algorithms. Later in the chapter, we will learn how to build a gradient agreement algorithm from scratch.
Chapter 9, Recent Advancements and Next Steps, starts by explaining task-agnostic meta learning, and then we will see how meta learning is used in an imitation learning setting. Then, we will learn how we can apply MAML in an unsupervised learning setting using the CACTUs algorithm. Then, we will explore a deep meta learning algorithm called learning to learn in the concept space.