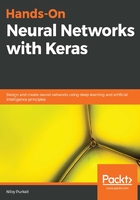
Building a network
The first architectural constraints that you must consider while building a network with dense layers are its the depth and width. Then, you need to define an input layer with the appropriate shape, and successively choose from different activation functions to use per layer.
As we did for our MNIST example, we simply import the sequential model and the dense layer structure. Then we proceed by initializing an empty sequential model and progressively add hidden layers until we reach the output layer. Do note that our input layer always requires a specific input shape, which for us corresponds to the 12,000 - dimensional one-hot encoded vectors that we will be feeding it. In our current model, the output layer only has one neuron, which will ideally fire if the sentiment in a given review is positive; otherwise, it won't. We will choose Rectified Linear Unit (ReLU) activation functions for our hidden layers and a sigmoid activation function for the ultimate layer. Recall that the sigmoid activation function simply squished probability values between 0 and 1, making it quite ideal for our binary classification task. The ReLU activation function simply helps us zero out negative values, and hence can be considered a good default to begin with in many deep learning tasks. In summary, we have chosen a model with three densely interconnected hidden layers, containing 18, 12, and 4 neurons, respectively, as well as an output layer with 1 neuron:
from keras.models import sequential
from keras.layers import Dense
model=Sequential()
model.add(Dense(6, activation='relu', input_shape=(12000)))
model.add(Dense(6, activation='relu'))
model.add(Dense(1, activation='sigmoid'))