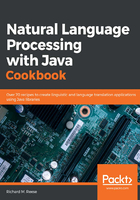
Performing SBD on specialized text
Unique types of text, such as medical or unusual languages, pose challenges when performing SBD. The frequent heavy use of specialized words and numeric values will not always yield good result with a model trained on a normal text. As a result, there are numerous models that have been trained on specialized datasets. In this recipe, we will demonstrate the use of a LingPipe model that has been trained to handle medical text.
The model will be demonstrated against an actual paragraph from a medical research article found in the Journal of Biomedical Science in 2018, Association between heavy metal levels and acute ischemic stroke, by Ching-Huang Lin, Yi-Ting Hsu, Cheng-Chung Yen, Hsin-Hung Chen, Ching-Jiunn Tseng, Yuk-Keung Lo, and Julie Y. H. Chan at https://jbiomedsci.biomedcentral.com/articles/10.1186/s12929-018-0446-0.
Specifically, we will use the paragraph found in the Results section of the article. It is shown here: