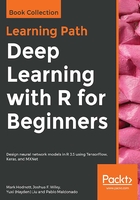
Neural networks in R
We will build several neural networks in this section. First, we will use the neuralnet package to create a neural network model that we can visualize. We will also use the nnet and RSNNS (Bergmeir, C., and Benítez, J. M. (2012)) packages. These are standard R packages and can be installed by the install.packages command or from the packages pane in RStudio. Although it is possible to use the nnet package directly, we are going to use it through the caret package, which is short for Classification and Regression Training. The caret package provides a standardized interface to work with many machine learning (ML) models in R, and also has some useful features for validation and performance assessment that we will use in this chapter and the next.
For our first examples of building neural networks, we will use the MNIST dataset, which is a classic classification problem: recognizing handwritten digits based on pictures. The data can be downloaded from the Apache MXNet site (https://apache-mxnet.s3-accelerate.dualstack.amazonaws.com/R/data/mnist_csv.zip). It is in the CSV format, where each column of the dataset, or feature, represents a pixel from the image. Each image has 784 pixels (28 x 28) and the pixels are in grayscale and range from 0 to 255. The first column contains the digit label, and the rest are pixel values, to be used for classification.