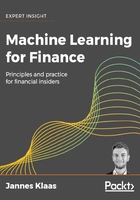
Chapter 2. Applying Machine Learning to Structured Data
Structured data is a term used for any data that resides in a fixed field within a record or file, two such examples being relational databases and spreadsheets. Usually, structured data is presented in a table in which each column presents a type of value, and each row represents a new entry. Its structured format means that this type of data lends itself to classical statistical analysis, which is also why most data science and analysis work is done on structured data.
In day-to-day life, structured data is also the most common type of data available to businesses, and most machine learning problems that need to be solved in finance deal with structured data in one way or another. The fundamentals of any modern company's day-to-day running is built around structured data, including, transactions, order books, option prices, and suppliers, which are all examples of information usually collected in spreadsheets or databases.
This chapter will walk you through a structured data problem involving credit card fraud, where we will use feature engineering to identify the fraudulent transaction from a dataset successfully. We'll also introduce the basics of an end-to-end (E2E) approach so that we can solve common financial problems.
Fraud is an unfortunate reality that all financial institutions have to deal with. It's a constant race between companies trying to protect their systems and fraudsters who are trying to defeat the protection in place. For a long time, fraud detection has relied on simple heuristics. For example, a large transaction made while you're in a country you usually don't live in will likely result in that transaction being flagged.
Yet, as fraudsters continue to understand and circumvent the rules, credit card providers are deploying increasingly sophisticated machine learning systems to counter this.
In this chapter, we'll look at how a real bank might tackle the problem of fraud. It's a real-world exploration of how a team of data scientists starts with a heuristic baseline, then develops an understanding of its features, and from that, builds increasingly sophisticated machine learning models that can detect fraud. While the data we will use is synthetic, the process of development and tools that we'll use to tackle fraud are similar to the tools and processes that are used every day by international retail banks.
So where do you start? To put it in the words of one anonymous fraud detection expert that I spoke to, "I keep thinking about how I would steal from my employer, and then I create some features that would catch my heist. To catch a fraudster, think like a fraudster." Yet, even the most ingenious feature engineers are not able to pick up on all the subtle and sometimes counterintuitive signs of fraud, which is why the industry is slowly shifting toward entirely E2E-trained systems. These systems, in addition to machine learning, are both focuses of this chapter where we will explore several commonly used approaches to flag fraud.
This chapter will act as an important baseline to Chapter 6, Using Generative Models, where we will again be revisiting the credit card fraud problem for a full E2E model using auto-encoders.