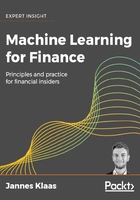
What this book covers
Chapter 1, Neural Networks and Gradient-Based Optimization, will explore what kinds of ML there are, and the motivations for using them in different areas of the financial industry. We will then learn how neural networks work and build one from scratch.
Chapter 2, Applying Machine Learning to Structured Data, will deal with data that resides in a fixed field within, for example, a relational database. We will walk through the process of model creation: from forming a heuristic, to building a simple model on engineered features, to a fully learned solution. On the way, we will learn about how to evaluate our models with scikit-learn, how to train tree-based methods such as random forests, and how to use Keras to build a neural network for this task.
Chapter 3, Utilizing Computer Vision, describes how computer vision allows us to perceive and interpret the real world at scale. In this chapter, we will learn the mechanisms with which computers can learn to identify image content. We will learn about convolutional neural networks and the Keras building blocks we need to design and train state-of-the-art computer vision models.
Chapter 4, Understanding Time Series, looks at the large number of tools devoted to the analysis of temporally related data. In this chapter, we will first discuss the "greatest hits" that industry professionals have been using to model time series and how to use them efficiently with Python. We will then discover how modern ML algorithms can find patterns in time series and how they are complemented by classic methods.
Chapter 5, Parsing Textual Data with Natural Language Processing, uses the spaCy library and a large corpus of news to discuss how common tasks such as named entity recognition and sentiment analysis can be performed quickly and efficiently. We will then learn how we can use Keras to build our own custom language models. The chapter introduces the Keras functional API, which allows us to build much more complex models that can, for instance, translate between languages.
Chapter 6, Using Generative Models, explains how generative models generate new data. This is useful when we either do not have enough data or want to analyze our data by learning about how the model perceives it. In this chapter, we will learn about (variational) autoencoders as well as generative adversarial models. We will learn how to make sense of them using the t-SNE algorithm and how to use them for unconventional purposes, such as catching credit card fraud. We will learn about how we can supplement human labeling operations with ML to streamline data collection and labeling. Finally, we will learn how to use active learning to collect the most useful data and greatly reduce data needs.
Chapter 7, Reinforcement Learning for Financial Markets, looks at reinforcement learning, which is an approach that does not require a human-labeled "correct" answer for training, but only a reward signal. In this chapter, we will discuss and implement several reinforcement learning algorithms, from Q-learning to Advantage Actor-Critic (A2C). We will discuss the underlying theory, its connection to economics, and in a practical example, see how reinforcement learning can be used to directly inform portfolio formation.
Chapter 8, Privacy, Debugging, and Launching Your Products, addresses how there is a lot that can go wrong when building and shipping complex models. We will discuss how to debug and test your data, how to keep sensitive data private while training models on it, how to prepare your data for training, and how to disentangle why your model is making the predictions it makes. We will then look at how to automatically tune your model's hyperparameters, how to use the learning rate to reduce overfitting, and how to diagnose and avoid exploding and vanishing gradients. After that, the chapter explains how to monitor and understand the right metrics in production. Finally, it discusses how you can improve the speed of your models.
Chapter 9, Fighting Bias, discusses how ML models can learn unfair policies and even break anti-discrimination laws. It highlights several approaches to improve model fairness, including pivot learning and causal learning. It shows how to inspect models and probe for bias. Finally, we discuss how unfairness can be a failure in the complex system that your model is embedded in and give a checklist that can help you reduce bias.
Chapter 10, Bayesian Inference and Probabilistic Programming, uses PyMC3 to discuss the theory and practical advantages of probabilistic programming. We will implement our own sampler, understand Bayes theorem numerically, and finally learn how we can infer the distribution of volatility from stock prices.