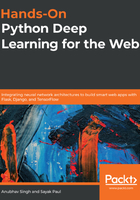
Web analytics
In the early years of the internet, many websites carried odometer-style counters embedded in them. These were simple counts of the number of hits the website or a particular page had received. Then, they grew in their available formats—plain counters, counters per day/week/month, and even geolocation-based counters.
The collection of data, which is essentially the logs of the interactions of users and how they interact with a web-based application, processing this data to produce performance indicators, and then finally to identify measures that can be taken by a company to improve their web application is collectively known as web analytics.
Since the invention of the internet, web applications today generate a huge amount of logs every moment. Even leaving your mouse pointer idle on a web page might be getting reported to a Google Analytics dashboard, from where the webmaster would be able to see which pages are being viewed by users and how much time they are spending on the pages. Also, the flow users take between pages would be a very interesting metric.
While the earliest web analytics tools would merely measure page hits, being able to create a map of how many times a given page was visited and how many times it was a unique user, they could hardly provide anything about the visiting patterns of users, unless they were specifically hardcoded, which would be presented in very generalized manners and were never website specific. The same form of analytics was being provided to a company doing e-commerce as was being provided to a personal website.
With the revolution that AI brought around in the web analytics domain, tools today that deploy the power of artificial intelligence can come up with future predictions of website performance and even suggest removing or adding specific content on a web page to improve user engagement with that page.