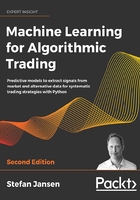
The baseline model – multiple linear regression
We will begin with the model's specification and objective function, the methods we can use to learn its parameters, and the statistical assumptions that allow the inference and diagnostics of these assumptions. Then, we will present extensions that we can use to adapt the model to situations that violate these assumptions. Useful references for additional background include Wooldridge (2002 and 2008).
How to formulate the model
The multiple regression model defines a linear functional relationship between one continuous outcome variable and p input variables that can be of any type but may require preprocessing. Multivariate regression, in contrast, refers to the regression of multiple outputs on multiple input variables.
In the population, the linear regression model has the following form for a single instance of the output y, an input vector , and the error
:

The interpretation of the coefficients is straightforward: the value of a coefficient is the partial, average effect of the variable xi on the output, holding all other variables constant.
We can also write the model more compactly in matrix form. In this case, y is a vector of N output observations, X is the design matrix with N rows of observations on the p variables plus a column of 1s for the intercept, and is the vector containing the P = p+1 coefficients:

The model is linear in its p +1 parameters but can represent nonlinear relationships if we choose or transform variables accordingly, for example, by including a polynomial basis expansion or logarithmic terms. You can also use categorical variables with dummy encoding, and include interactions between variables by creating new inputs of the form xixj.
To complete the formulation of the model from a statistical point of view so that we can test hypotheses about its parameters, we need to make specific assumptions about the error term. We'll do this after introducing the most important methods to learn the parameters.
How to train the model
There are several methods we can use to learn the model parameters from the data: ordinary least squares (OLS), maximum likelihood estimation (MLE), and stochastic gradient descent (SGD). We will present each method in turn.
Ordinary least squares – how to fit a hyperplane to the data
The method of least squares is the original method that learns the parameters of the hyperplane that best approximates the output from the input data. As the name suggests, it takes the best approximation to minimize the sum of the squared distances between the output value and the hyperplane represented by the model.
The difference between the model's prediction and the actual outcome for a given data point is the residual (whereas the deviation of the true model from the true output in the population is called error). Hence, in formal terms, the least-squares estimation method chooses the coefficient vector to minimize the residual sum of squares (RSS):

Thus, the least-squares coefficients are computed as:

The optimal parameter vector that minimizes the RSS results from setting the derivatives with respect to of the preceding expression to zero. Assuming X has full column rank, which requires that the input variables are not linearly dependent, it is thus invertible, and we obtain a unique solution, as follows:

When y and X have means of zero, which can be achieved by subtracting their respective means, represents the ratio of the covariance between the inputs and the outputs
and the output variance
There is also a geometric interpretation: the coefficients that minimize RSS ensure that the vector of residuals is orthogonal to the subspace of
spanned by the P columns of X, and the estimates
are orthogonal projections into that subspace.
Maximum likelihood estimation
MLE is an important general method used to estimate the parameters of a statistical model. It relies on the likelihood function, which computes how likely it is to observe the sample of outputs when given the input data as a function of the model parameters. The likelihood differs from probabilities in that it is not normalized to a range from 0 to 1.
We can set up the likelihood function for the multiple linear regression example by assuming a distribution for the error term, such as the standard normal distribution:

This allows us to compute the conditional probability of observing a given output yi given the corresponding input vector xi and the parameters ,
:

Assuming the output values are conditionally independent, given the inputs, the likelihood of the sample is proportional to the product of the conditional probabilities of the inpidual output data points. Since it is easier to work with sums than with products, we apply the logarithm to obtain the log-likelihood function:

The goal of MLE is to choose the model parameters that maximize the probability of the observed output sample, taking the inputs as given. Hence, the MLE parameter estimate results from maximizing the log-likelihood function:

Due to the assumption of normally distributed errors, maximizing the log-likelihood function produces the same parameter solution as least squares. This is because the only expression that depends on the parameters is the squared residual in the exponent.
For other distributional assumptions and models, MLE will produce different results, as we will see in the last section on binary classification, where the outcome follows a Bernoulli distribution. Furthermore, MLE is a more general estimation method because, in many cases, the least-squares method is not applicable, as we will see later for logistic regression.
Gradient descent
Gradient descent is a general-purpose optimization algorithm that will find stationary points of smooth functions. The solution will be a global optimum if the objective function is convex. Variations of gradient descent are widely used in training complex neural networks, but also to compute solutions for MLE problems.
The algorithm uses the gradient of the objective function. The gradient contains the partial derivatives of the objective with respect to the parameters. These derivatives indicate how much the objective changes for an infinitesimal (infinitely small) step in the direction of the corresponding parameters. It turns out that the maximal change of the function value results from a step in the direction of the gradient itself.
Figure 7.1 sketches the process for a single variable x and a convex function f(x), where we are looking for the minimum, x0 . Where the function has a negative slope, gradient descent increases the target value for x0, and decreases the values otherwise:

Figure 7.1: Gradient descent
When we minimize a function that describes, for example, the cost of a prediction error, the algorithm computes the gradient for the current parameter values using the training data. Then, it modifies each parameter in proportion to the negative value of its corresponding gradient component. As a result, the objective function will assume a lower value and move the parameters closer to the solution. The optimization stops when the gradient becomes small, and the parameter values change very little.
The size of these steps is determined by the learning rate, which is a critical parameter that may require tuning. Many implementations include the option for this learning rate to gradually decrease with the number of iterations. Depending on the size of the data, the algorithm may iterate many times over the entire dataset. Each such iteration is called an epoch. The number of epochs and the tolerance used to stop further iterations are additional hyperparameters you can tune.
Stochastic gradient descent randomly selects a data point and computes the gradient for this data point, as opposed to an average over a larger sample to achieve a speedup. There are also batch versions that use a certain number of data points for each step.
The Gauss–Markov theorem
To assess the statistical properties of the model and run inference, we need to make assumptions about the residuals that represent the part of the input data the model is unable to correctly fit or "explain."
The Gauss–Markov theorem (GMT) defines the assumptions required for OLS to produce unbiased estimates of the model parameters , and for these estimates to have the lowest standard error among all linear models for cross-sectional data.
The baseline multiple regression model makes the following GMT assumptions (Wooldridge 2008):
- In the population, linearity holds so that
, where
are unknown but constant and
is a random error.
- The data for the input variables
is a random sample from the population.
- No perfect collinearity—there are no exact linear relationships among the input variables.
- The error
has a conditional mean of zero given any of the inputs:
.
- Homoskedasticity—the error term
has constant variance given the inputs:
The fourth assumption implies that no missing variable exists that is correlated with any of the input variables.
Under the first four assumptions (GMT 1-4), the OLS method delivers unbiased estimates. Including an irrelevant variable does not bias the intercept and slope estimates, but omitting a relevant variable will result in biased parameter estimates.
Under GMT 1-4, OLS is then also consistent: as the sample size increases, the estimates converge to the true value as the standard errors become arbitrary. The converse is, unfortunately, also true: if the conditional expectation of the error is not zero because the model misses a relevant variable or the functional form is wrong (for example, quadratic or log terms are missing), then all parameter estimates are biased. If the error is correlated with any of the input variables, then OLS is also not consistent and adding more data will not remove the bias.
If we add the fifth assumption, then OLS also produces the best linear unbiased estimates (BLUE). Best means that the estimates have the lowest standard error among all linear estimators. Hence, if the five assumptions hold and the goal is statistical inference, then the OLS estimates are the way to go. If the goal, however, is to predict, then we will see that other estimators exist that trade some bias for a lower variance to achieve superior predictive performance in many settings.
Now that we have introduced the basic OLS assumptions, we can take a look at inference in small and large samples.
How to conduct statistical inference
Inference in the linear regression context aims to draw conclusions from the sample data about the true relationship in the population. This includes testing hypotheses about the significance of the overall relationship or the values of particular coefficients, as well as estimates of confidence intervals.
The key ingredient for statistical inference is a test statistic with a known distribution, typically computed from a quantity of interest like a regression coefficient. We can formulate a null hypothesis about this statistic and compute the probability of observing the actual value for this statistic, given the sample under the assumption that the hypothesis is correct. This probability is commonly referred to as the p-value: if it drops below a significance threshold (typically 5 percent), then we reject the hypothesis because it makes the value that we observed for the test statistic in the sample very unlikely. On the flip side, the p-value reflects the probability that we are wrong in rejecting what is, in fact, a correct hypothesis.
In addition to the five GMT assumptions, the classical linear model assumes normality—that the population error is normally distributed and independent of the input variables. This strong assumption implies that the output variable is normally distributed, conditional on the input variables. It allows for the derivation of the exact distribution of the coefficients, which, in turn, implies exact distributions of the test statistics that are needed for exact hypotheses tests in small samples. This assumption often fails in practice—asset returns, for instance, are not normally distributed.
Fortunately, however, the test statistics used under normality are also approximately valid when normality does not hold. More specifically, the following distributional characteristics of the test statistics hold approximately under GMT assumptions 1–5 and exactly when normality holds:
- The parameter estimates follow a multivariate normal distribution:
.
- Under GMT 1–5, the parameter estimates are unbiased, and we can get an unbiased estimate of
, the constant error variance, using
.
- The t-statistic for a hypothesis test about an inpidual coefficient
is
and follows a t distribution with N-p-1 degrees of freedom, where
is the j's element of the diagonal of
.
- The t distribution converges to the normal distribution. Since the 97.5 quantile of the normal distribution is about 1.96, a useful rule of thumb for a 95 percent confidence interval around a parameter estimate is
, where se means standard error. An interval that includes zero implies that we can't reject the null hypothesis that the true parameter is zero and, hence, irrelevant for the model.
- The F-statistic allows for tests of restrictions on several parameters, including whether the entire regression is significant. It measures the change (reduction) in the RSS that results from additional variables.
- Finally, the Lagrange multiplier (LM) test is an alternative to the F-test for testing multiple restrictions.
How to diagnose and remedy problems
Diagnostics validate the model assumptions and help us prevent wrong conclusions when interpreting the result and conducting statistical inference. They include goodness of fit measures and various tests of the assumptions about the error term, including how closely the residuals match a normal distribution.
Furthermore, diagnostics evaluate whether the residual variance is indeed constant or exhibits heteroskedasticity (covered later in this section). They also test if the errors are conditionally uncorrelated or exhibit serial correlation, that is, if knowing one error helps to predict consecutive errors.
In addition to conducting the following diagnostic tests, you should always visually inspect the residuals. This helps to detect whether they reflect systematic patterns, as opposed to random noise that suggests the model is missing one or more factors that drive the outcome.
Goodness of fit
Goodness-of-fit measures assess how well a model explains the variation in the outcome. They help to evaluate the quality of the model specification, for instance, when selecting among different model designs.
Goodness-of-fit metrics differ in how they measure the fit. Here, we will focus on in-sample metrics; we will use out-of-sample testing and cross-validation when we focus on predictive models in the next section.
Prominent goodness-of-fit measures include the (adjusted) R2, which should be maximized and is based on the least-squares estimate:
- R2 measures the share of the variation in the outcome data explained by the model and is computed as
, where TSS is the sum of squared deviations of the outcome from its mean. It also corresponds to the squared correlation coefficient between the actual outcome values and those estimated by the model. The implicit goal is to maximize R2. However, it never decreases as we add more variables. One of the shortcomings of R2, therefore, is that it encourages overfitting.
- The adjusted R2 penalizes R2 for adding more variables; each additional variable needs to reduce the RSS significantly to produce better goodness of fit.
Alternatively, the Akaike information criterion (AIC) and the Bayesian information criterion (BIC) are to be minimized and are based on the maximum-likelihood estimate:
, where
is the value of the maximized likelihood function and k is the number of parameters.
, where N is the sample size.
Both metrics penalize for complexity. BIC imposes a higher penalty, so it might underfit relative to AIC and vice versa.
Conceptually, AIC aims to find the model that best describes an unknown data-generating process, whereas BIC tries to find the best model among the set of candidates. In practice, both criteria can be used jointly to guide model selection when the goal is an in-sample fit; otherwise, cross-validation and selection based on estimates of generalization error are preferable.
Heteroskedasticity
GMT assumption 5 requires the residual covariance to take the shape , that is, a diagonal matrix with entries equal to the constant variance of the error term. Heteroskedasticity occurs when the residual variance is not constant but differs across observations. If the residual variance is positively correlated with an input variable, that is, when errors are larger for input values that are far from their mean, then OLS standard error estimates will be too low; consequently, the t-statistic will be inflated, leading to false discoveries of relationships where none actually exist.
Diagnostics starts with a visual inspection of the residuals. Systematic patterns in the (supposedly random) residuals suggest statistical tests of the null hypothesis that errors are homoscedastic against various alternatives. These tests include the Breusch–Pagan and White tests.
There are several ways to correct OLS estimates for heteroskedasticity:
- Robust standard errors (sometimes called White standard errors) take heteroskedasticity into account when computing the error variance using a so-called sandwich estimator.
- Clustered standard errors assume that there are distinct groups in your data that are homoscedastic, but the error variance differs between groups. These groups could be different asset classes or equities from different industries.
Several alternatives to OLS estimate the error covariance matrix using different assumptions when . The following are available in statsmodels:
- Weighted least squares (WLS): For heteroskedastic errors where the covariance matrix has only diagonal entries, as for OLS, but now the entries are allowed to vary.
- Feasible generalized least squares (GLSAR): For autocorrelated errors that follow an autoregressive AR(p) process (see Chapter 9, Time-Series Models for Volatility Forecasts and Statistical Arbitrage).
- Generalized least squares (GLS): For arbitrary covariance matrix structure; yields efficient and unbiased estimates in the presence of heteroskedasticity or serial correlation.
Serial correlation
Serial correlation means that consecutive residuals produced by linear regression are correlated, which violates the fourth GMT assumption. Positive serial correlation implies that the standard errors are underestimated and that the t-statistics will be inflated, leading to false discoveries if ignored. However, there are procedures to correct for serial correlation when calculating standard errors.
The Durbin–Watson statistic diagnoses serial correlation. It tests the hypothesis that the OLS residuals are not autocorrelated against the alternative that they follow an autoregressive process (which we will explore in the next chapter). The test statistic ranges from 0 to 4; values near 2 indicate non-autocorrelation, lower values suggest positive autocorrelation, and higher values indicate negative autocorrelation. The exact threshold values depend on the number of parameters and observations and need to be looked up in tables.
Multicollinearity
Multicollinearity occurs when two or more independent variables are highly correlated. This poses several challenges:
- It is difficult to determine which factors influence the dependent variable.
- The inpidual p-values can be misleading—a p-value can be high, even if the variable is, in fact, important.
- The confidence intervals for the regression coefficients will be too wide, possibly even including zero. This complicates the determination of an independent variable's effect on the outcome.
There is no formal or theory-based solution that corrects for multicollinearity. Instead, try to remove one or more of the correlated input variables, or increase the sample size.