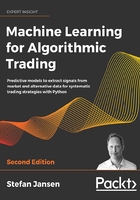
上QQ阅读APP看书,第一时间看更新
Alpha factor resources
The research process requires designing and selecting alpha factors with respect to the predictive power of their signals. An algorithmic trading strategy will typically build on multiple alpha factors that send signals for each asset. These factors may be aggregated using an ML model to optimize how the various signals translate into decisions about the timing and sizing of inpidual positions, as we will see in subsequent chapters.
Alternative algorithmic trading libraries
Additional open source Python libraries for algorithmic trading and data collection include the following (see GitHub for links):
- QuantConnect is a competitor to Quantopian.
- WorldQuant offers online competition and recruits community contributors to a crowd-sourced hedge fund.
- Alpha Trading Labs offers an s high-frequency focused testing infrastructure with a business model similar to Quantopian.
- The Python Algorithmic Trading Library (PyAlgoTrade) focuses on backtesting and offers support for paper trading and live trading. It allows you to evaluate an idea for a trading strategy with historical data and aims to do so with minimal effort.
- pybacktest is a vectorized backtesting framework that uses pandas and aims to be compact, simple, and fast. (The project is currently on hold.)
- ultrafinance is an older project that combines real-time financial data collection and the analysis and backtesting of trading strategies.
- Trading with Python offers courses and a collection of functions and classes for quantitative trading.
- Interactive Brokers offers a Python API for live trading on their platform.