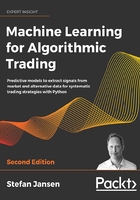
Summary
In this chapter, we introduced new sources of alternative data made available as a result of the big data revolution, including inpiduals, business processes, and sensors, such as satellites or GPS location devices. We presented a framework to evaluate alternative datasets from an investment perspective and laid out key categories and providers to help you navigate this vast and quickly expanding area that provides critical inputs for algorithmic trading strategies that use ML.
We also explored powerful Python tools you can use to collect your own datasets at scale. We did this so that you can potentially work on getting your private informational edge as an algorithmic trader using web scraping.
We will now proceed, in the following chapter, to the design and evaluation of alpha factors that produce trading signals and look at how to combine them in a portfolio context.