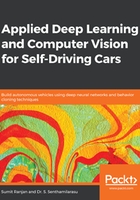
Dive Deep into Deep Neural Networks
In this chapter, you will learn about a topic that has changed the way we think about autonomous driving: Artificial Neural Networks (ANNs). Throughout this chapter, you will learn how these algorithms can be used to build a self-driving car perception stack, and you'll learn about the different components needed to design and train a deep neural network. This chapter will teach you everything you need to know about ANNs. You will also learn about the building blocks of feedforward neural networks, a very useful basic type of ANN. Specifically, we'll look at the hidden layers of a feedforward neural network. These hidden layers are important as they differentiate the mode of action of neural networks from the rest of the Machine Learning (ML) algorithms. We'll begin by looking at the mathematical definition of feedforward neural networks so that you can start to understand how to build these algorithms for the perception stacks of self-driving cars.
Before ML became popular for object detection, we used to use the Histogram of Oriented Gradients (HOG) and classifiers. The main goal was to train a model that recognizes the shapes of an object by recognizing its different gradients or its orientation. HOG retains the shape and direction of the images, it counts occurrences of the gradient in a localized portion of the image.
In recent years, Deep Learning (DL) has become very popular for its performance due to advances in computational power, the arrival of powerful Graphical Processor Units (GPUs), and its ability to accumulate more data. Before GPUs, it was difficult to process deep learning algorithms on our machines, so a big reason for recent developments is cheaper computation.
We will start this chapter with an introduction to neural networks and deep learning. We will also learn about neurons, the activation function, the cost function, and hyperparameters. We will implement our first deep learning model in Chapter 3, Implementing a Deep Learning Model Using Keras.
In this chapter, we will cover the following topics:
Diving deep into neural networks
Understanding neurons and perceptrons
The workings of ANNs
Understanding activation functions
The cost function of neural networks
Optimizers
Understanding hyperparameters
TensorFlow versus Keras