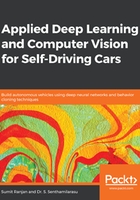
Introduction to SDCs
The following is an image of an SDC by WAYMO undergoing testing in Los Altos, California:
You can check out the image at https://en.wikipedia.org/wiki/File:Waymo_Chrysler_Pacifica_in_Los_Altos,_2017.jpg.
The idea of the autonomous car has existed for decades, but we saw enormous improvement from 2002 onward when the Defense Advanced Research Projects Agency (DARPA) announced the first of its grand challenges, called the DARPA Grand Challenge (2004). That would forever change the world's perception of what autonomous robots can do. The first event was held in 2004 and DARPA offered the winners a one-million-dollar prize if they could build an autonomous vehicle that was able to navigate 142 miles through the Mojave Desert. Although the first event saw only a few teams get off the start line (Carnegie Mellon's red team took first place, having driven only 7 miles), it was clear that the task of driving without any human aid was indeed possible. In the second DARPA Grand Challenge in 2005, five of the 23 teams smashed expectations and successfully completed the track without any human intervention at all. Stanford's vehicle, Stanley, won the challenge, followed by Carnegie Mellon's Sandstorm, an autonomous vehicle. With this, the era of driverless cars had arrived.
Later, the 2007 installment, called the DARPA Urban Challenge, invited universities to show off their autonomous vehicles on busy roads with professional stunt drivers. This time, after a harrowing 30-minute delay that occurred due to a jumbotron screen blocking their vehicle from receiving GPS signals, the Carnegie Mellon team came out on top, while the Stanford Junior vehicle came second.
Collectively, these three grand challenges were truly a watershed moment in the development of SDCs, changing the way the public (and more importantly, the technology and automotive industries) thought about the feasibility of full vehicular autonomy. It was now clear that a massive new market was opening up, and the race was on. Google immediately brought in the team leads from both Carnegie Mellon and Stanford (Chris Thompson and Mike Monte-Carlo, respectively) to push their designs onto public roads. By 2010, Google's SDC had logged over 140 thousand miles in California, and they later wrote in a blog post that they were confident about cutting the number of traffic deaths by half using SDCs. blog by Google: What we're driving at — Sebastian Thrun (https://googleblog.blogspot.com/2010/10/what-were-driving-at.html)
As per a study released by the Virginia Tech Transportation Institute (VTTI) and the National Highway Traffic Safety Administration (NHTSA), 80% of car accidents involve human distraction (https://seriousaccidents.com/legal-advice/top-causes-of-car-accidents/driver-distractions/). An SDC can, therefore, become a useful and safe solution for the whole of society to reduce these accidents. In order to propose a path that an intelligent car should follow, we require several software applications to process data using artificial intelligence (AI).
Google succeeded in creating the world's first autonomous car 2 years ago (at the time of writing). The problem with Google's car was its expensive 3D RADAR, which is worth about $75,000.
The solution to this cost is to use multiple, cheaper cameras that are mounted to the car to capture images that recognize the lane lines on the road, as well as the real-time position of the car.
In addition, a driverless car can reduce the distance between cars, thereby reducing the degree of road loads, reducing the number of traffic jams. Furthermore, they greatly reduce the capacity for human errors to occur while driving and allow people with disabilities to drive long distances.
A machine as a driver will never make a mistake; it will be able to calculate the distance between cars very accurately. Parking will be more efficiently spaced, and the fuel consumption of cars will be optimized.
The driverless car is a vehicle equipped with sensors and cameras for detecting the environment, and it can navigate (almost) without any real-time input from a human. Many companies are investing billions of dollars in order to advance this toward an accessible reality. Now, a world where AI takes control of driving has never been closer.
Nowadays, self-driving car engineers are exploring several different approaches in order to develop an autonomous system. The most successful and popularly used among them are as follows:
The robotics approach
The deep learning approach
In reality, in the development of SDCs, both robotics and deep learning methods are being actively pursued by developers and engineers.
The robotic approach works by fusing output from a set of sensors to analyze a vehicle's environment directly and prompt it to navigate accordingly. For many years, self-driving automotive engineers have been working on and improving robotic approaches. However, more recently, engineering teams have started developing autonomous vehicles using a deep learning approach.
Deep neural networks enable SDCs to learn how to drive by imitating the behavior of human driving.
The five core components of SDCs are computer vision, sensor fusion, localization, path planning, and control of the vehicle.
In the following diagram, we can see the five core components of SDCs:
Let's take a brief look at these core components:
Computer vision can be considered the eyes of the SDC, and it helps us figure out what the world around it looks like.
Sensor fusion is a way of incorporating the data from various sensors such as RADAR, LIDAR, and LASER to gain a deeper understanding of our surroundings.
Once we have a deeper understanding of what the world around it looks like, we want to know where we are in the world, and localization helps with this.
After understanding what the world looks like and where we are in the world, we want to know where we would like to go, so we use path planning to chart the course of our travel. Path planning is built for trajectory execution.
Finally, control helps with turning the steering wheel, changing the car's gears, and applying the brakes.
Getting the car to autonomously follow the path you want requires a lot of effort, but researchers have made the possible with the help of advanced systems engineering. Details regarding systems engineering will be provided later in this chapter.
Benefits of SDCs
Indeed, some people may be afraid of autonomous driving, but it is hard to deny its benefits. Let's explore a few of the benefits of autonomous vehicles:
Greater safety on roads: Government data identifies that a driver's error is the cause of 94% of crashes (https://crashstats.nhtsa.dot.gov/Api/Public/ViewPublication/812115). Higher levels of autonomy can reduce accidents by eliminating driver errors. The most significant outcome of autonomous driving could be to reduce the devastation caused by unsafe driving, and in particular, driving under the influence of drugs or alcohol. Furthermore, it could reduce the heightened risks for unbelted occupants of vehicles, vehicles traveling at higher speeds, and distractions that affect human drivers. SDCs will address these issues and increase safety, which we will see in more detail in the Levels of autonomy section of this chapter.
Greater independence for those with mobility problems: Full automation generally offers us more personal freedom. People with special needs, particularly those with mobility limitations, will be more self-reliant. People with limited vision who may be unable to drive themselves will be able to access the freedom afforded by motorized transport. These vehicles can also play an essential role in enhancing the independence of senior citizens. Furthermore, mobility will also become more affordable for people who cannot afford it as ride-sharing will reduce personal transportation costs.
Reduced congestion: Using SDCs could address several causes of traffic congestion. Fewer accidents will mean fewer backups on the highway. More efficient, safer distances between vehicles and a reduction in the number of stop-and-go waves will reduce the overall congestion on the road.
Reduced environmental impact: Most autonomous vehicles are designed to be fully electric, which is why the autonomous vehicle has the potential to reduce fuel consumption and carbon emissions, which will save fuel and reduce greenhouse gas emissions from unnecessary engine idling.
There are, however, potential disadvantages to SDCs:
The loss of vehicle-driving jobs in the transport industry as a direct impact of the widespread adoption of automated vehicles.
Loss of privacy due to the location and position of an SDC being integrated into an interface. If it can be accessed by other people, then it can be misused for any crime.
A risk of automotive hacking, particularly when vehicles communicate with each other.
The risk of terrorist attacks also exists; there is a real possibility of SDCs, charged with explosives, being used as remote car bombs.
Despite these disadvantages, automobile companies, along with governments, need to come up with solutions to the aforementioned issues before we can have fully automated cars on the roads.
Advancements in SDCs
The idea of SDCs on our streets seemed like a crazy sci-fi fantasy until just a few years ago. However, the rapid progress made in recent years in both AI and autonomous technology proves that the SDC is becoming a reality. But while this technology appears to have emerged virtually overnight, it has been a long and winding path to achieving the self-driving vehicles of today. In reality, it wasn't long after the invention of the motor car when inventors started thinking about autonomous vehicles.
In 1925, former US army electrical engineer and founder of The Houdina Radio Control Co., Francis P Houdina, developed a radio-operated automobile. He equipped a Chandler motor car with a transmitting antenna and operated it from a second car that followed it using a transmitter.
In the early 1990s, Dean Pomerleau, who is a PhD researcher from Carnegie Mellon University, did something interesting in the field of SDCs. Firstly, he described how neural networks could allow an SDC to take images of the road and predict steering control in real time. Then, in 1995, along with his fellow researcher Todd Jochem, he drove an SDC that they created on the road. Although their SDC required driver control of the speed and brakes, it traveled around 2,797 miles.
Then came the grand challenge by DARPA in 2002, which we discussed previously. This competition offered a $1 million prize to any researcher who could build a driverless vehicle. It was stipulated that the vehicle should be able to navigate 142 miles through the Mojave Desert. The challenge kicked off in 2004, but none of the competitors were able to complete the course. The winning team traveled for less than 8 miles in a couple of hours.
In the early 2000s, when the autonomous car was still futuristic, self-parking systems began to evolve. Toyota's Japanese Prius hybrid vehicle started offering automatic parking assistance in 2003. This was later followed by BMW and Ford in 2009.
Google secretly started an SDC project in 2009. The project was initially led by Sabastian Thrun, the former director of the Stanford Artificial Intelligence Laboratory and co-inventor of Google Street View. The project is now called Waymo. In August 2012, Google revealed that their driverless car had driven 300,000 miles without a single accident occurring.
Since the 1980s, various companies such as General Motors, Ford, Mercedes-Benz, Volvo, Toyota, and BMW have started working on their own autonomous vehicles. As of 2019, 29 US states have passed legislation enabling autonomous vehicles.
Nvidia Xavier is an SDC chip that has incorporated AI capabilities. Nvidia also announced a collaboration with Volkswagen to transform this dream into a reality, by developing AI for SDCs.
On this journey to becoming a reality, the driverless car has launched into a frenzied race that includes tech companies and start-ups, as well as traditional automakers.
The market for self-driving vehicles, including cars and trucks, is categorized into transport and defense, based on their application. Transportation is expected to emerge in the future which is further divided into industrial, commercial, and consumer applications.
The SDC and self-driving truck market size is estimated to grow to 6.7 per thousand vehicular units globally in 2020 and is expected to increase at a compound annual growth rate (CAGR) of 63.1% from 2021 to 2030.
It is expected that the highest adoption of driverless vehicles will be in the US due to the increase in government support for the market gateway of the autonomous vehicle. The US transportation secretary Elaine Chao signaled strong support for SDCs in the CES tech conference, Las Vegas, which was organized by the Consumer Technology Association on January 7th, 2020.
Additionally, it is expected that Europe will also emerge as a potentially lucrative market for technological advancements in self-driving vehicles with increasing consumer preference.
In the next section, we will learn about the challenges in current deployments of autonomous driving.