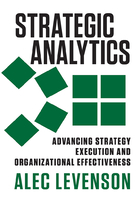
Chapter 1
Of Elephants and Incomplete Analytics
Issues Addressed in This Chapter
• Most analytics conducted today by the business and by HR are incomplete and cannot solve strategy execution problems on their own
• You need a full causal model to diagnose the entire system and to understand what really drives behavior and performance
Key Questions
• What problems are you trying to solve with your analysis?
• How does the analysis you have chosen help to improve strategy execution?
You need to know what drives performance in your organization to get strategy execution right. The problem with organizational analytics today is that they tell an incomplete story. Enterprise analytics and human capital analytics are conducted along parallel and separate tracks. Both attempt to determine why performance happens, yet each on its own can tell only part of the story. Without the complete story, we don’t really know the best ways to improve strategy execution and organizational effectiveness.
Enterprise analytics can tell us if we’re achieving the strategy and details about the operational measures that contribute to strategy execution. A typical analysis addresses questions like these:
• What types of customers can best help increase our share in existing markets?
• What new markets can we succeed in?
• What organizational capabilities do we need for strategic success?
The enterprise metrics used to answer these questions include market share, sales, and margins, among others, and extend to operational and technical measures that describe business processes, such as productivity, innovation, quality, manufacturing uptime, time-to-market, customer service, and others.
On the human capital side, a typical analysis tries to figure out the sources of organizational ineffectiveness, focusing almost exclusively on how work is done and whether people work well together. A typical analysis addresses questions like these:
• Who are our best leaders, and what role do they really play?
• Is a group or team performing well or working at cross purposes?
• What is the right mix of compensation versus non-monetary rewards in motivating performance?
• How can we improve our HR practices to be more effective?
The human capital metrics used to answer these questions include leadership and frontline worker competencies, employee attitudes, and measures of human resource (HR) program efficiency and effectiveness.
Enterprise analytics tells us whether the strategy is executed. But it can’t tell us which jobs and individual-level behaviors most directly lead to improved strategy execution. The human capital perspective is essential, but only on rare occasions are people from the human capital side invited to contribute when senior leaders conduct business analytics. Instead, they usually are told what happened only after the key decisions were made.
Business analytics and HR analytics as commonly practiced are examples of incomplete analysis: they do not specify and test a full causal model. Rather than looking at the entire system that defines and drives organizational performance, they take shortcuts and focus on too few elements. To illustrate the problem with the analysis of large complex systems based on only partial data, consider the scene in figure 3, which is from a centuries-old fable.

Figure 3: The Perils of Incomplete Data Analysis
The scene is a group of blind monks who are each touching different parts of an elephant. Modern versions of the story star blind men, and sometimes only three of them, though the specific stars of the story are not important. What matters is the analysis performed by each person, which occurs in isolation and without consideration of the data gathered by the other people.
WHAT’S THE RIGHT LEVEL OF CUSTOMER SERVICE? A SYSTEMS DIAGNOSIS APPROACH.
Business-to-consumer industries. Determining the right amount of customer service is a challenge for all organizations. If you don’t provide enough, key customers walk out the door. If you provide too much, profit margins get whittled down to nothing and you don’t make any money.
Customer service in business-to-consumer industries is driven by product quality, ease of use, and responsiveness of customer service representatives (CSRs). When customer service scores fall, the directive to operational leaders may simply be “Go figure out how to get customer service back to where it was before.” Suppose product development previously decided to save costs by reducing spending on quality assurance processes. They may have assessed that existing processes are redundant, slowing time to market and reducing sales. If the lower spending on quality assurance is misguided, the end result would be lower product quality and unhappy customers. If the customer service scores do not measure product quality, fingers could be pointed at the CSRs, leading to the incorrect conclusion that they had become less motivated to provide high-quality customer service.
For another case, consider the link between CSR compensation and customer service. Suppose the customer service site leader is held accountable for metrics such as time to resolve customer complaints and efficiency of the operations (call volume, wait times, and similar issues). If she does not have profit and loss (P&L) responsibility for her operations she will push for higher compensation for her CSRs as a way to attract and retain higher skilled employees. Similarly, HR might advocate for greater pay to reduce attrition and improve retention of the longer-tenured and more experienced CSRs. However, evaluating whether better pay is worth the investment requires a complete Strategic Analytics diagnostic that addresses the relationship between customer retention and sales and profitability.
Business-to-business industries. In business-to-business industries, customer service involves striking the right balance between cutting prices to make the sale and maximizing profits. A Strategic Analytics diagnostic looks at the complete set of interactions between the customer and organization, along with the role played by each employee and function. For example, salespeople may be given discretion to set specific contract terms, but they need timely and accurate information on how the terms impact enterprise profitability through metrics such as capacity utilization. And they need to be trained and evaluated on overall profitability, not just sales.
The person touching the tail concludes that an elephant has features like a rope. The person touching the leg thinks the elephant’s shape is more like a tree. The person touching the tusk has no idea what the elephant’s skin feels like. And so on. All their conclusions seem reasonable, given the information at each person’s disposal, but all fail to describe the entire animal properly.
Enterprise analytics today are like the person touching only the head. They are out in front, focusing on a part of the animal that is pointed forward. Yet trying to dictate the direction and pace of the animal by focusing only the head can be a lost cause. If the animal’s legs are tethered to a post, it cannot move, no matter what you do. You may point the animal in the right direction, but you will never get it to move forward.
Human capital analytics today are like the person touching only the hind legs. They move in unison with other parts of the body, but they contribute only one part to the animal’s full range of motion and have no effect on direction. You can’t properly diagnose problems with overall direction and speed by ignoring the rest of the animal and analyzing just the rear legs.
What’s missing from both types of analysis is the rest of the body: the front legs that work in unison with the rear legs to propel the body forward and the torso that holds it all together. Excluding the trunk leaves out key information about how the animal maintains its health through eating, drinking, and bathing. Enterprise and human capital analytics, when conducted separately, fall short of identifying and testing a complete causal model. The most accurate insights require a combined analysis that diagnoses the performance drivers for the entire system, not just one part of it.
Data mining is not causal analysis. One of the biggest mistakes I see frequently is simple data analysis that focuses on only one or two pieces of information. The problem is that simple analysis without a causal model can lead to the wrong conclusions: it is just data mining and not science. A typical example is when consultants look at a group of companies and conclude that “the use of HR practice XYZ is more common at high-performing companies,” where XYZ could be the latest leadership development program, incentive pay philosophy, employee engagement strategy, and so on. The implication is that if your organization adopts the same practice, your business results will improve. The implicit causal model is shown in figure 4.
The problem is that HR practices by themselves do not create business results. They are one contribution in a larger system that enables the business results. HR practices can improve strategy execution only when they are aligned with other parts of the system.

Figure 4: An Overly Simple Causal Model of HR Practices and Strategy Execution
Consider the following incomplete analysis example. To start, let’s say that customer retention drops, triggering alarm bells. A quick scan of available data might reveal that at approximately the same time there was an increase in turnover among customer service agents. Putting those two pieces of information side by side, many people would conclude that the increase in agent turnover “caused” the drop in customer retention. But consider some alternative explanations and data:
• What if the increase in turnover was concentrated among low-performing agents? In this case the turnover might have been caused by the drop in customer retention, not the other way around, as managers attempted to address low retention through improved customer service.
• What if there was a recent change in the managerial team at a big call center where many agents work? A new leadership team might be inexperienced and unable to maintain previous levels of both customer satisfaction and employee engagement, leading both to fall simultaneously.
• What if a new customer relationship management (CRM) system was recently introduced? Problems with getting a new system to work properly could drive both customers and employees to leave.
• What if the external labor market for agents has been heating up in recent months? Failure to keep up with the market in terms of pay, benefits, and developmental opportunities can drive turnover.
• What if there has been a steady decline in employee engagement scores on the annual survey? Longer-term trends in employee wellbeing could either be unrelated to the recent loss of customers—or a direct contributor to it.
Each of these alternatives is a hypothesis about the relationship between customer retention and employee turnover. Each hypothesis was formed by introducing additional pieces of information to the original empirical observation that customer retention and employee turnover are statistically related. The problem is that each piece of data on its own is not enough to test these hypotheses. A Strategic Analytics causal model is needed to combine all relevant information together for joint testing.

Figure 5: A Causal Model of Human Capital and Enterprise Performance
Figure 5 presents a causal model you can use for the Strategic Analytics diagnostic. The model is used throughout the book to show how to conduct integrated analytics. It features two main parts: human capital (or individual) performance in the top half and enterprise (or organizational) performance in the bottom half, with the arrows indicating casual pathways. Individual capability, job design (roles and responsibilities), and attitudes (motivation) combine to produce human capital performance. Enterprise performance occurs when people in their individual roles do their jobs according to the organization design, creating the organizational capability through their actions, with culture acting as a catalyst.
For most strategic objectives in almost all industries, successful execution requires the combined efforts of multiple people in different roles working together as a team. That means considering both the top and bottom parts of the causal model in figure 5.
For some roles in organizations, the top part of the causal model in figure 5 is all that is needed. These roles directly contribute to strategy execution with minimal reliance on or interdependence with other roles. For example, if individual salespeople can (almost) singlehandedly increase sales, then the top part of the causal model may be sufficient to analyze sales performance. If the objective of your strategy is customer satisfaction and retention, then the top part of the causal model may also be sufficient for customer service agents. Other examples that focus primarily on human capital performance with little to no emphasis on enterprise performance are covered in chapter 7’s case studies on retaining critical talent (figure 12) and evaluating the impact of executive coaching (figure 13).
Group, team, site, business unit, or organizational performance all can be addressed with the bottom part of the causal model in figure 5. The contribution of all roles together within the context of culture creates the task execution for the group, which in turn leads to the business results (strategy execution). In some cases, you can focus the analytics exclusively at the enterprise level and pay little to no attention to the human capital level. Examples from chapter 7 include the case studies on speed of decision making (figure 11) and role redundancy.
Examples where both in-depth enterprise and human capital analysis are needed include chapter 7’s case studies on route sales maximization (figure 14) and business unit optimization (figure 15). These require more time and are harder to conduct than the other two types—analysis primarily only at the human capital or only at the enterprise levels. Which type of analysis you choose to conduct, though, needs to be driven by the information you need for a proper systems diagnosis, not by expediency.
Figure 5 is simultaneously simple yet powerful. It is simple enough that it can be used to describe how business performance can be achieved for virtually any strategic objective or organizational process. It is powerful because the multiple levels of analysis can yield the critical insights needed to boost performance and improve execution of your strategy. For example, consider the hypotheses about the link between customer retention and customer service agent turnover from earlier in this chapter:
• Increased turnover of low-performing agents means an increase in average agent capability, a factor in the upper left part of the model.
• A recent change in the managerial team at a big call center means a change in the organizational support the agents at that call center receive, which could show up as a change in attitudes and behaviors at the individual level and/or culture and behaviors at the group (call center) levels.
• Problems implementing a new customer relationship management (CRM) system, a change in the job design, could show up as either an increased or decreased ability to perform the job tasks.
• More outside employment opportunities would make it harder to attract and retain high-quality agents.
• A steady decline in employee engagement scores means worse attitudes.
Each individual piece of data that was used to try and determine a link between customer service agent turnover and customer retention represents one or more elements of the causal model in figure 5. Focusing on only one piece of data at a time just because it “makes sense” or “feels right” is no substitute for building the complete causal model. Though the hypotheses derived from individual pieces of data may all be reasonable in isolation, you need integrated analysis to determine the true causes of strategy execution.
In certain situations, like some of the case studies presented in chapter 7, you won’t end up doing extensive analysis at one of the two levels—either at the enterprise level or at the human capital level. However, you need the first step in Strategic Analytics to reliably determine if you can focus primarily on only one of those two levels. If competitive advantage analytics show that it’s sufficient to do extensive analysis only at one of the two levels, that’s fine. In contrast, skipping over step 1 and focusing on only enterprise or human capital analytics just because you are pressed for time or because you’re not familiar with the other approach is not.
Part II addresses how to do the analysis. But before we get to that, chapter 2 takes a look at ROI and other common analysis tools. Their deficiencies highlight the value of doing Strategic Analytics.